Significance of Water Quality Prediction and AI
Heatwaves and disruptions in water cycle systems driven by climate change are increasingly impacting the water quality (WQ) of inland waters. Ensuring the maintenance of WQ is critical for both environmental sustainability and effective water resource management. Traditional methods, such as monitoring WQ indicators (e.g., chlorophyll-a, dissolved oxygen, total phosphorus, total nitrogen, water temperature) through WQ observatories are often labor-intensive and limited in spatial and temporal coverage.
Our Goal
We aim to develop a robust AI-based prediction model to estimate chlorophyll-a concentrations in inland lakes and rivers. By enhancing prediction accuracy and expanding spatial coverage, this project aims to strengthen efforts to monitor and address water pollution, eutrophication, and other critical environmental issues.
Our Approach
Our approach integrates high-resolution satellite images (e.g., Landsat-8/9 and Sentinel-2/3, etc) with key environmental variables from land surface models (e.g, ERA5 Land, GLDAS and MERRA-2, etc). The model accounts for the distinct characteristics of lakes and rivers, enabling customized solutions for each water body type.
Future research plans include incorporating socio-statistical data, such as population density and livestock metrics, as well as climate change scenarios (e.g., RCP and SSP). This will allow us to develop predictive models that account for the influence of human activities and long-term climate dynamics.
By expanding the range of data inputs and leveraging AI's predictive capabilities, we aim to provide a comprehensive solution for understanding and mitigating the impacts of climate change on the WQ of inland waters.
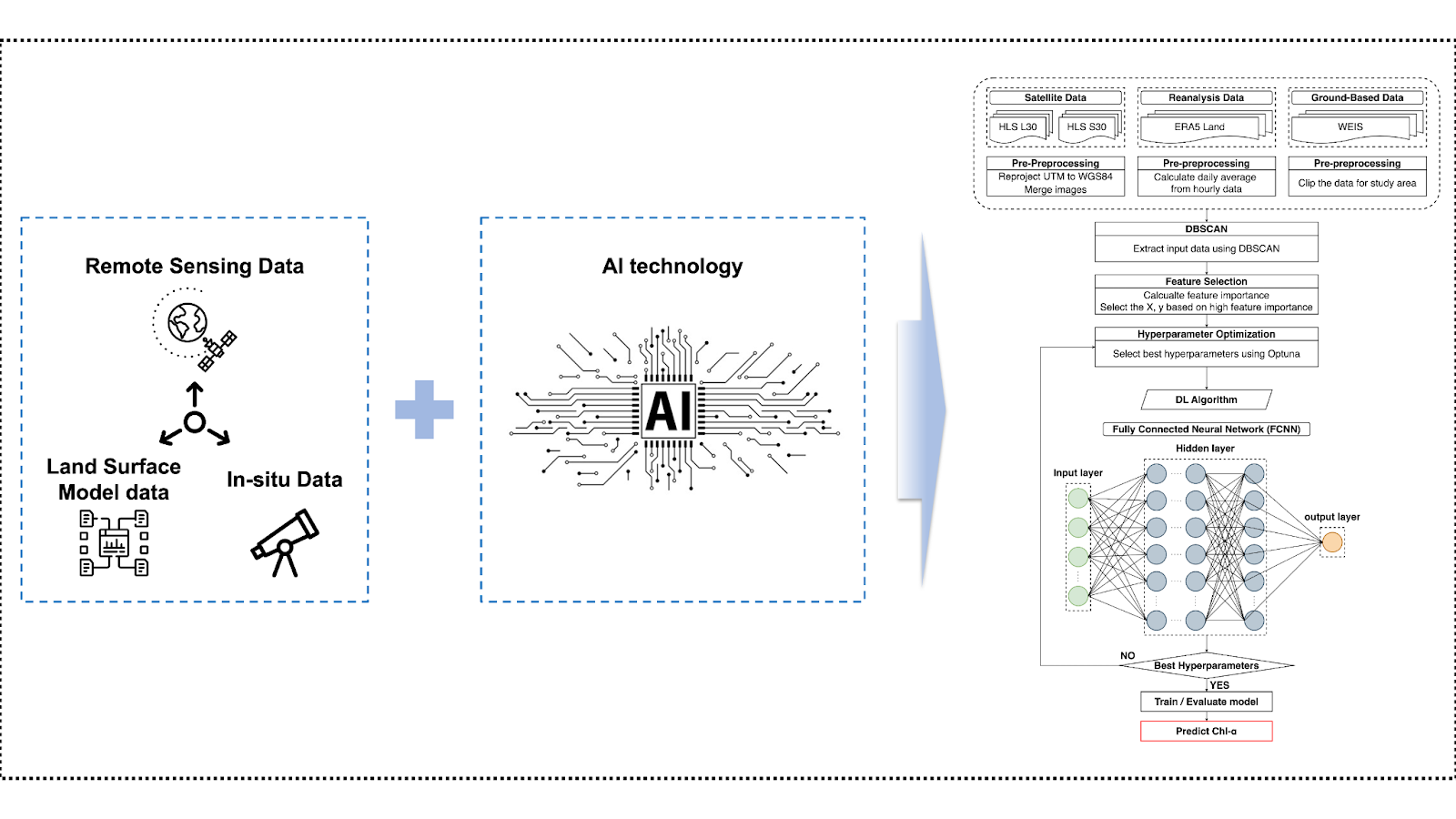
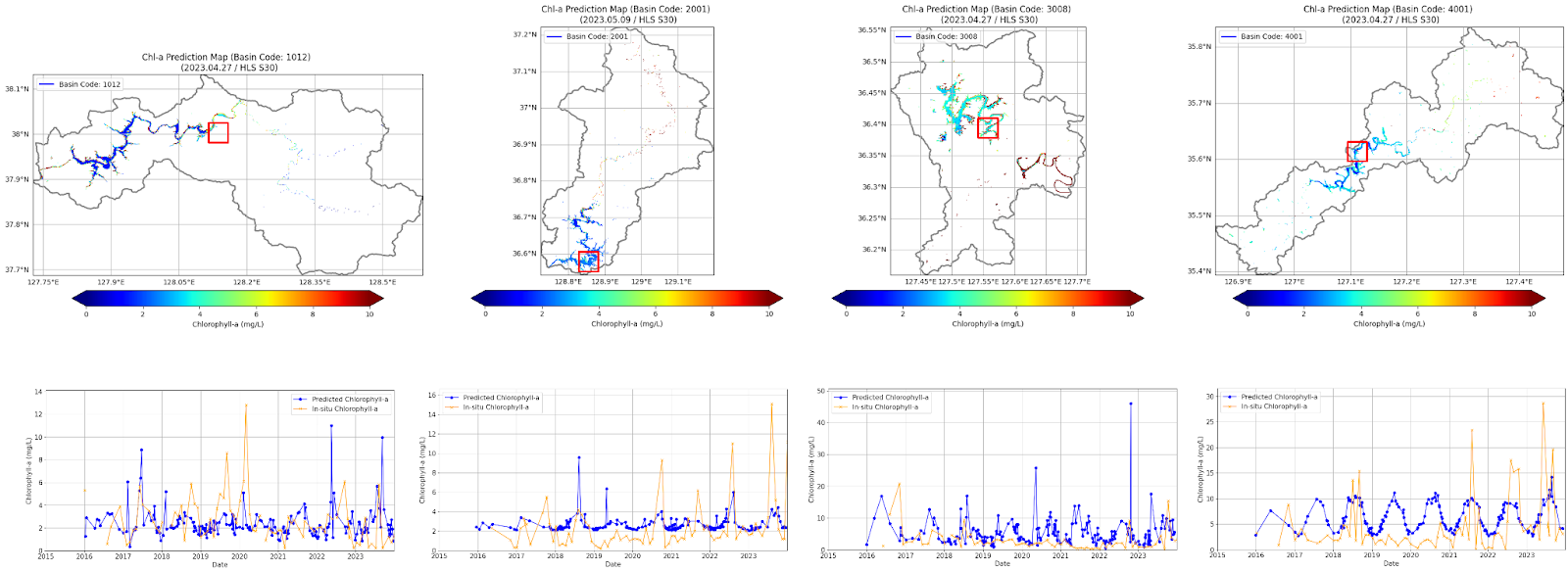
Tools and Expertise
Our research combines a variety of data sources, technologies, and domain expertise:
Programming: Python for data processing, model training, and visualization.
Spatial Analysis: GIS tools for mapping and spatial data interpretation.
Computing: High-performance computing to process and analyze large datasets.
Domain Knowledge: Expertise in hydrology and environmental science for interpreting results.
Key Areas of Research Interest
In addition to chlorophyll-a prediction, our project explores the following topics:
- Investigating AI approaches for low-concentration chlorophyll-a prediction in rivers.
- Analyzing the impact of environmental factors (e.g., precipitation, flow speed) on WQ.
- Developing transfer learning methods for regional and global inland water prediction.
- Exploring multi-sensor data fusion to enhance model accuracy and scalability.
- Incorporating uncertainty quantification into prediction models for decision-making.
- Extending the methodology to other WQ indicators like turbidity and dissolved oxygen.