Improving Earth System Predictions: Assimilating Satellite-Based Observations with Ensemble Kalman Filter and Machine Learning Techniques
Data assimilation is an advanced method used in Earth sciences to combine observational data, such as those obtained from satellites, with model simulations to generate more accurate and reliable forecasts. It bridges the gap between real-world measurements and theoretical models, allowing scientists to gain a deeper understanding of various Earth systems. In our research lab, we focus on assimilating satellite-based observations of soil moisture, vegetation, and groundwater into a land surface model. To achieve this, we employ the Ensemble Kalman Filter, a sophisticated statistical technique that adjusts model predictions based on real-time data. Additionally, we utilize machine learning techniques to further refine our model, ensuring that our simulations are as accurate and reliable as possible for better decision-making in environmental management and climate adaptation.
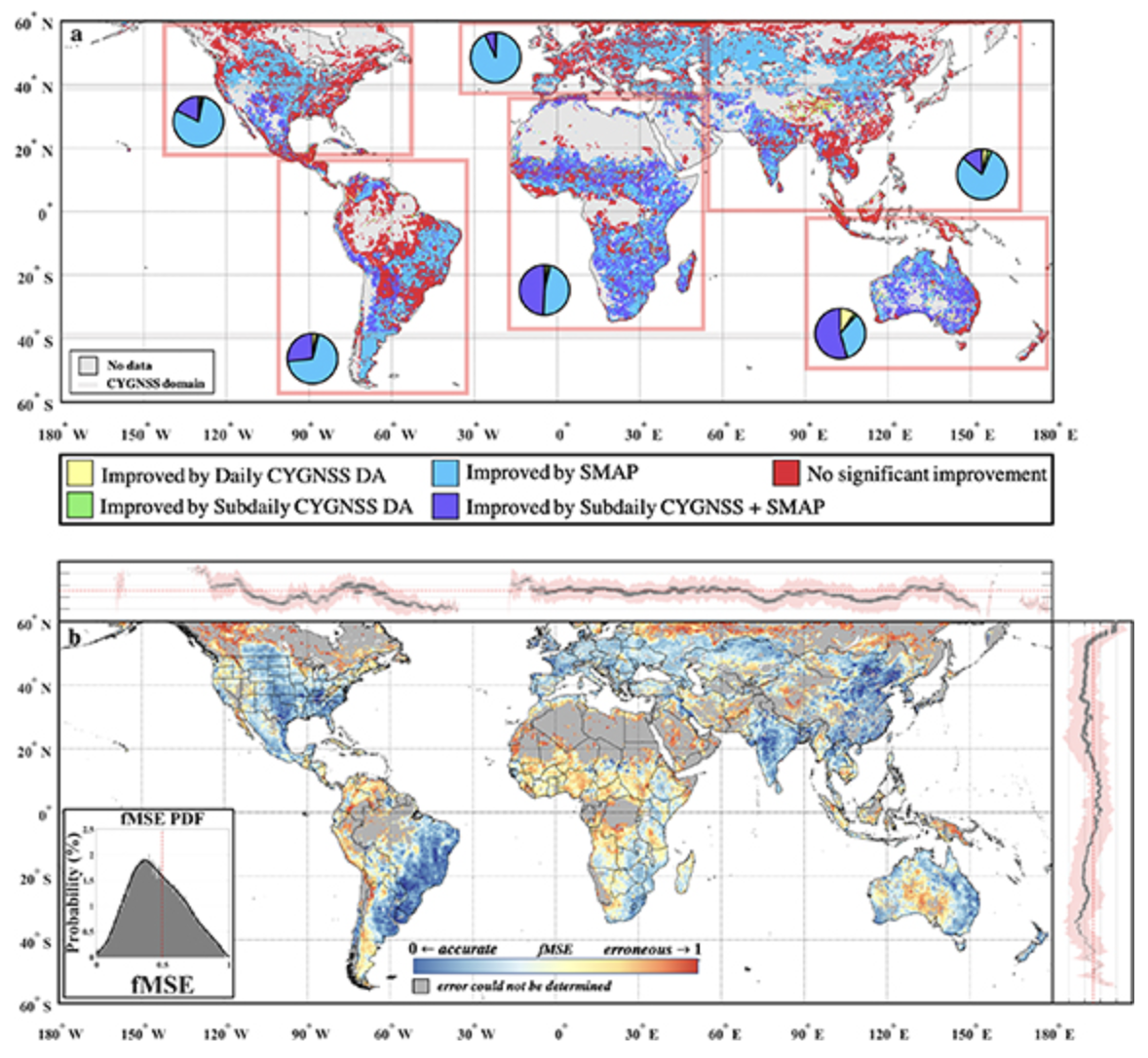
What we aim to achieve
Through data assimilation, we aim to achieve more accurate and reliable representations of Earth systems, ultimately improving predictions and forecasts for natural disasters such as drought, flood, dust outbreaks, and wildfires. By integrating diverse observational data with model simulations, we can enhance the understanding of complex processes, allowing for better decision-making in environmental management, climate adaptation, natural resource planning, and disaster risk reduction.
Data and analytic skills we use for this project
To perform data assimilation, individuals need a strong foundation in various data and analytic skills, including:
- Mathematics and Statistics: A solid understanding of probability theory, linear algebra, and statistical methods is crucial for interpreting and analyzing data in data assimilation.
- Numerical Methods: Familiarity with numerical methods, such as interpolation and optimization, is essential for working with large datasets and implementing assimilation algorithms.
- Programming: Proficiency in programming languages like Python, R, MATLAB, or Fortran is necessary for data manipulation, model implementation, and analysis.
- Earth System Models: Knowledge of the specific Earth system model being used (e.g., land surface) is vital for understanding the processes and variables involved in the assimilation process.
- Remote Sensing and GIS: Skills in remote sensing, GIS, and spatial data analysis are important for working with satellite observations and incorporating them into models.
- Data Management: Ability to work with large datasets, manage data storage, and handle various data formats (e.g., NetCDF, HDF) is crucial for efficient data assimilation.
- Machine Learning and Artificial Intelligence: Familiarity with machine learning techniques and AI algorithms can enhance the assimilation process, providing more advanced methods for model improvement and prediction.
- Domain Knowledge: Understanding the specific Earth system domain (e.g., hydrology) ensures accurate interpretation of results and proper application of data assimilation techniques.
We can learn together and we are here to help you develop these skills.
This project aims not only to assimilate satellite data sets into land surface models but also to explore other related research topics below. If you are interested in any of the following research areas, please do not hesitate to contact me!
- Multi-source Data Assimilation: Investigate the integration of diverse data sources, such as ground-based observations, satellite remote sensing, and citizen science data, to improve Earth system models.
- Data Assimilation in Urban Environments: Study the use of data assimilation techniques to improve urban-scale environmental models, addressing issues like air pollution, heat islands, and urban flooding.
- Deep Learning for Data Assimilation: Investigate the potential of deep learning algorithms, such as neural networks, for enhancing data assimilation processes and improving Earth system model predictions.
- Uncertainty Quantification in Data Assimilation: Develop novel methods to assess and reduce uncertainties in data assimilation, focusing on both observational data and model predictions.
- Ensemble-based Data Assimilation Techniques: Explore the development and application of advanced ensemble-based data assimilation methods, such as particle filters or ensemble-based variational methods, to improve Earth system models.
- Data Assimilation in Climate Change Projections: Study the potential of data assimilation to reduce uncertainties in climate change projections and improve the accuracy of future climate scenarios.
- Real-time Data Assimilation for Disaster Management: Explore the use of data assimilation techniques for real-time monitoring and forecasting of natural disasters, such as hurricanes, earthquakes, and wildfires, to support early warning systems and disaster risk reduction efforts.