Importance of Machine Learning in Microwave Soil Moisture Data Disaggregation
Microwave soil moisture data from satellite sensors provide crucial information for agriculture, weather forecasting, and climate modeling, but the spatial resolution of these data is often coarse. Disaggregation using machine learning techniques can improve the spatial resolution of these data, providing more detailed information on soil moisture conditions at a local scale. Machine learning algorithms can also learn complex relationships between microwave signals and soil moisture content, which can be difficult to model using traditional techniques.
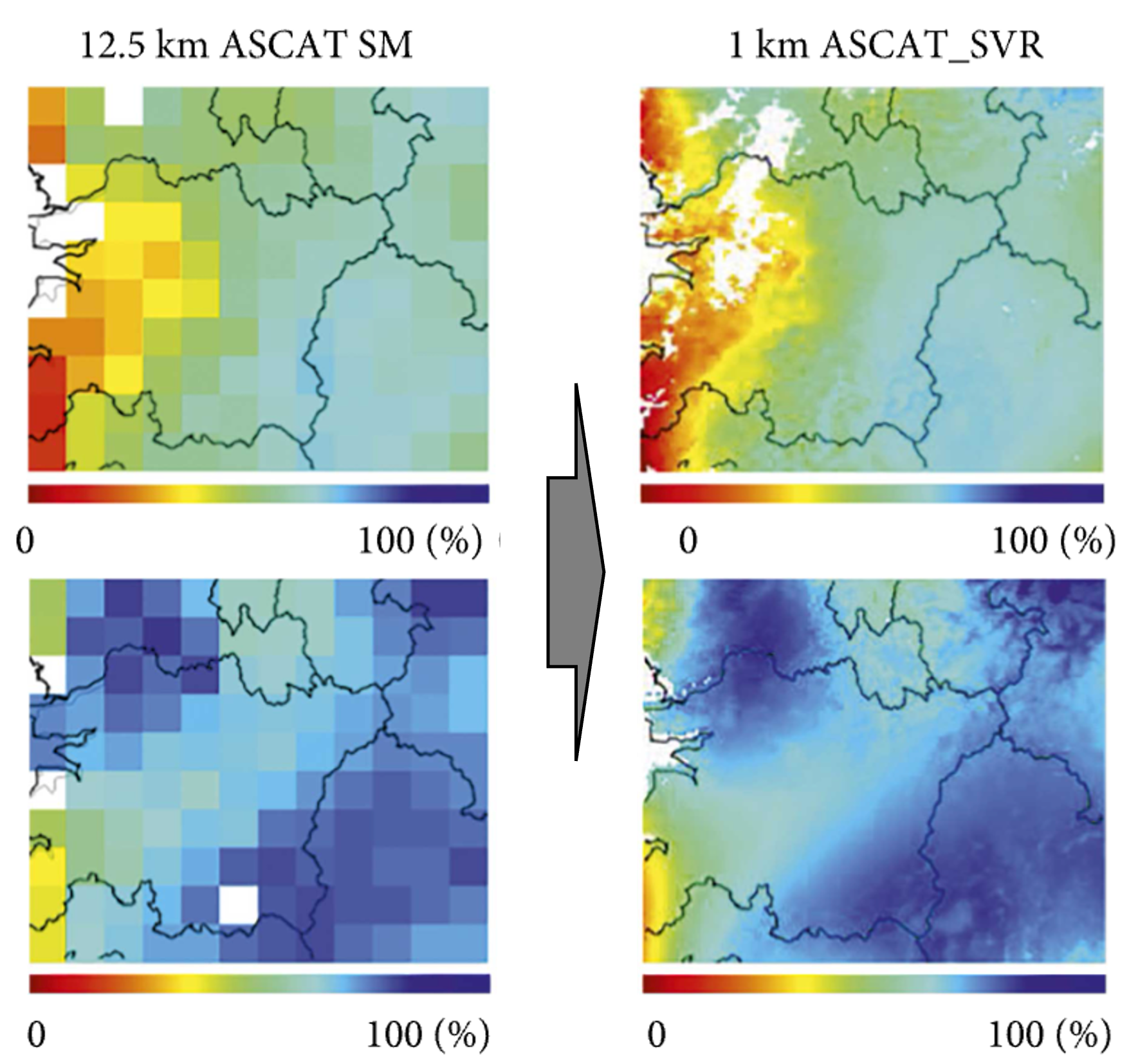
What we aim to achieve
We aim to achieve higher spatial resolution and more accurate estimates of soil moisture conditions through soil moisture data disaggregation with various machine learning algorithms. This can help improve our understanding and prediction of droughts, floods, and other weather-related events, as well as aid in agriculture and water resource management.
We are disaggregating soil moisture data using machine learning algorithms, which are trained on a combination of high-resolution soil moisture data and low-resolution microwave satellite data. The algorithm learns the relationship between the two data sets and then applies this relationship to the low-resolution microwave data to estimate soil moisture at a higher resolution. Some common machine learning algorithms used for this task include neural networks, random forests, and support vector machines. Additionally, we can also use auxiliary data such as topography, vegetation, and soil properties to further improve the accuracy of the disaggregated soil moisture data.
Data and analytic skills we use for this project
- Satellite remote sensing data from various Earth observing satellites including GCOM-W2, Landsat family, and microwave satellite systems
- Machine learning algorithms, such as neural networks, random forests, and support vector machines
- Python or R programming for data processing, analysis, and visualization
- GIS (Geographic Information System) software for spatial data analysis and mapping
- High-performance computing skills for processing large datasets
- Knowledge of soil science and hydrology to interpret and validate results
This project aims not only to disaggregate the satellite-based soil moisture data but also to explore other related research topics below. If you are interested in any of the following research areas, please do not hesitate to contact me!
- Investigating the effectiveness of different machine learning algorithms for soil moisture data disaggregation, including deep learning models.
- Developing new feature extraction techniques to improve the accuracy of soil moisture estimates.
- Exploring the use of multi-sensor data fusion techniques to improve the resolution and accuracy of soil moisture data.
- Investigating the transferability of machine learning models across different regions and climatic zones.
- Developing methods for incorporating uncertainty information into disaggregated soil moisture data.
- Investigating the impact of different preprocessing techniques, such as smoothing and denoising, on the accuracy of soil moisture estimates.
- Developing methods for integrating high-resolution soil moisture data from ground-based sensors with low-resolution satellite data.
- Exploring the use of other microwave satellite data, such as synthetic aperture radar (SAR), for soil moisture data disaggregation.
- Developing methods for estimating soil moisture profiles using machine learning techniques.
- Investigating the use of disaggregated soil moisture data for improving hydrological modeling and water resource management.